Researchers at ETH University in Zurich, Switzerland are using reinforcement learning on a robot platform, enabling it to adapt to data it collects.
The team, from the university’s autonomous systems lab, is working to create a platform that autonomously operates in complex environments and learns from processes that it fulfils.
Using a Clearpath Robotics’ Ridgeback robot platform as a base, the team added a Franka Emika Panda manipulator arm and nicknamed the technology RoyalPanda.
RoyalPanda is used to experiment with a series of different scenarios to develop best practices of how a robot could ‘learn’.
Researcher Julien Kindle said: “The Clearpath Ridgeback platform was very handy from the beginning in the creation of a simulation, to the end in deployment of the real system, thanks to its out-of-the-box ROS compatibility.
“That way, we were able to focus on our work instead of having to develop our own drivers and models for ROS.”
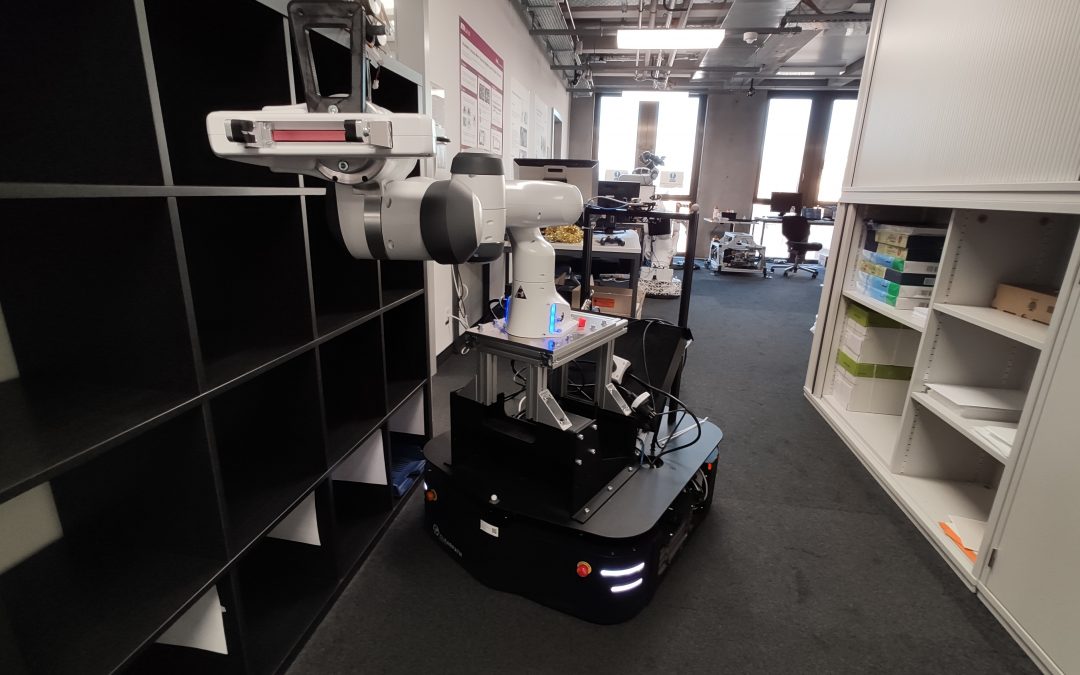
The team is focussing on the mechatronic design and control of RoyalPanda, which is designed to autonomously adapt to different situations and cope with uncertain and dynamic daily environments.
The researchers are interested in developing robot concepts from real-world testing on land, air and on water. They will also work with methods and tools for perception, abstraction, mapping, and path planning.
The project aims to explore the possibilities and limitations of using reinforcement learning to train a neural network. It will work in a simulation that controls all degrees of freedom of a mobile manipulator – i.e. joints of the manipulator and movement of the base platform – before deploying it on a real robot.
Initially, the team worked with a robot that was constrained to move in one plane and directly fed data from two 2D lidar scans to the network. This gave the controlling agent an understanding of its environment.
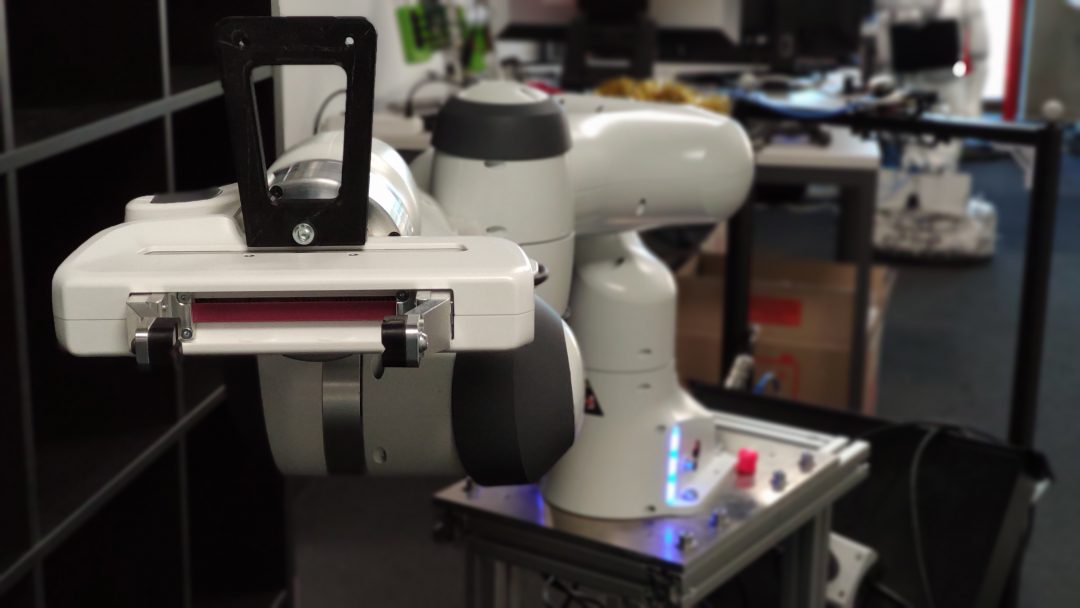
Currently the researchers are working to deal with core flaws such as restricted obstacle avoidance capabilities. The project has designed its own approach that lays the foundation of a controller that overcomes these problems while keeping the computational demand very low, therefore being capable of running in real-time on low-end devices.
Additionally, the team said it was able to deploy a reinforcement learning agent in the form of a neural network that was trained in simulation to a real robot in a variety of corridor environments.
The project said it slowly increase the complexity of the simulation to improve the speed and robustness of convergence, thereby giving the agent a better understanding of a real-world scenario.
The team aims to use RoyalPanda in future projects and has also created similar combinations including RoyalYumi. Featuring Ridgeback with an ABB YuMi cobot, this combination can fetch and carry applications in unstructured indoor environments.