Coventry’s Manufacturing Technology Centre has developed a flexible industrial robot with decision-making capabilities similar to a human operator.
The team of robotics engineers used a combination of machine-learning and visual recognition to teach the robot, which combines a robot operating system with a collaborative robot and vision sensors, to make assembly decisions based on the components put in front of it.
According to MTC, this is a breakthrough development that could save manufacturers the costs of expensive fixed tooling.
MTC has created a flexible automation demonstrator to show manufacturers how the robot can be used to create a low-cost, reactive assembly system.
It mimics a typical electronic assembly using multiple components and is trained to recognise components and assembly variables as well as retrieve solutions from its database.
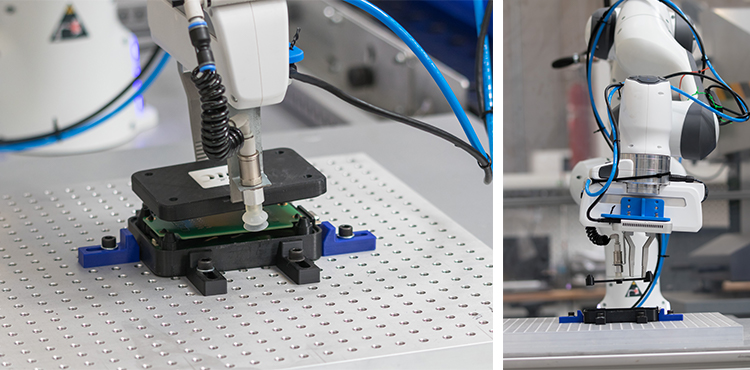
MTC senior research engineer Mark Robson said: “Giving robots the decision-making capability of a human operator can dramatically improve their productivity and flexibility in variable conditions.
“Our demonstrator project shows how machine learning can be applied to achieve this.
“This work has shown that deep learning-based vision can provide robots with a robust ability to find and work with objects.
“The MTC has demonstrated methods to overcome the challenges in translating this ability into the physical domain of robotics which will enable the use of other machine-learning algorithms in industrial solutions.”
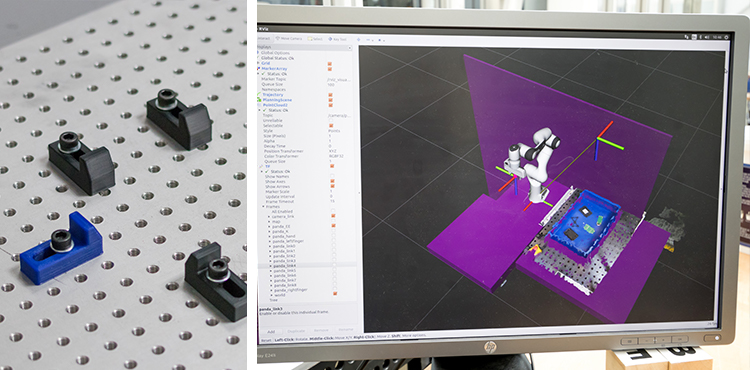
During trials the MTC system returned a 99% successful detection rate and demonstrated that it was possible to swap the input tray and change the component mix with little effect on performance.
MTC’s chief automation officer, Mike Wilson, said: “We now have a blueprint for developing and implementing intelligent vision systems to industrial problems in an effective way, opening up potential applications in human-robot collaboration.
“We have eliminated the need for part fixtures as this system can adapt to new layouts without reprogramming.
“Completely traceable decision-making allows errors to be addressed quickly and effectively, and the system can be trained to apply to any new assembly process.”